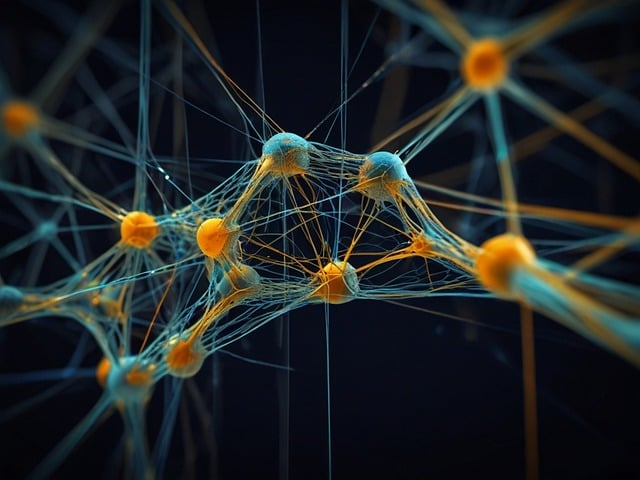
In the realm of artificial intelligence, generative AI stands out as a transformative force, propelling innovations in various sectors from creative arts to sophisticated data analysis. On Day 2 of our series, we delve deep into the mechanics of generative AI, elucidating the intricate neural networks and machine learning algorithms that fuel this powerful technology. Let's embark on a technical exploration of Generative AI.
Understanding the Basics: Neural Networks
At the heart of generative AI are neural networks—computational systems inspired by the human brain's architecture. These networks consist of layers of interconnected nodes or "neurons," which process and transmit information. Each neuron receives input, performs a calculation and passes its output to subsequent neurons. The complexity and depth of these interactions define the network's ability to learn and generate outputs.
1. Structure: Neural networks typically comprise three layers: the input layer, hidden layers and the output layer. The input layer receives raw data, which is processed through one or more hidden layers that extract features and patterns. The output layer then produces the final result based on the learned data representations.
2. Learning Process: The process through which neural networks learn is called "training." During training, the network adjusts its internal parameters (weights and biases) based on the difference between its output and the actual data (the error). Techniques like backpropagation are used to minimize this error across numerous iterations, refining the network’s accuracy.
Diving Deeper: Machine Learning Algorithms
Generative AI leverages a subset of machine learning called "deep learning," which involves deeper neural networks capable of understanding data at multiple abstraction levels. Two pivotal architectures within generative AI are Generative Adversarial Networks (GANs) and Variational Autoencoders (VAEs).
1. Generative Adversarial Networks (GANs): A GAN consists of two competing neural network models—a generator and a discriminator. The generator creates data instances (like images), while the discriminator evaluates them against real data, guiding the generator to produce more authentic outputs. This adversarial process continues until the generator produces data indistinguishable from genuine data, thereby learning the distribution of input data.
2. Variational Autoencoders (VAEs): VAEs are designed to compress data into a lower-dimensional space and then reconstruct it back into its original form. They learn the parameters of a probability distribution representing the data, allowing them to generate new data points with similar properties by sampling from this distribution.
Applications and Implications
The applications of generative AI are vast and varied. In creative industries, it assists in designing art and music that mimic human creativity. In business, it can generate realistic simulations and models to aid decision-making processes. The technology's ability to understand and replicate complex data patterns also makes it invaluable in predictive analytics, enhancing everything from marketing strategies to healthcare prognostics.
Generative AI is not just a technical marvel; it is reshaping industries and redefining the boundaries of what machines can achieve. As we continue to explore and harness its potential, understanding its underlying mechanics will be crucial for innovators and leaders across fields.
Comments